ALGORITHMIC AUDITING
FOR
MUSIC DISCOVERABILITY [aa4md]
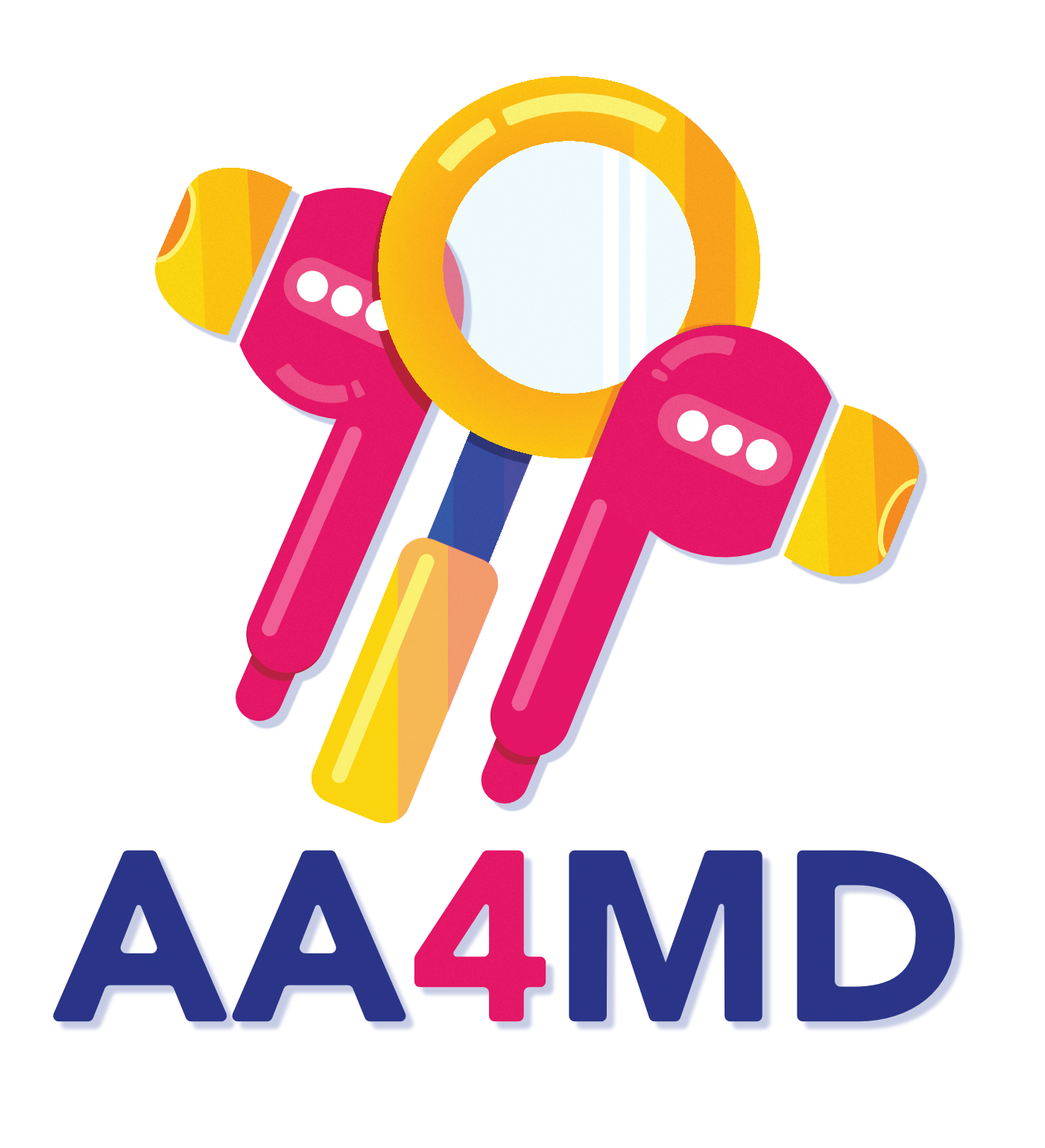
Auditing recommender systems — safeguarding discoverability of culturally diverse music
Algorithm audits are empirical studies investigating a public algorithmic system for potential problematic behaviour. They have emerged with the explosion of online platforms and AI applications to address the threat of biased outcomes, lack of transparency and misuse of data. With the support of the Marie Skłodowska-Curie Actions programme the AA4MD project aims to develop auditing techniques involving end users that are tailored specifically for recommender systems. The project will include the development of a web-based tool for large-scale audits as well as policy recommendations. Its overarching goal is to increase exposure to culturally diverse music.
The AA4MD project seeks to addressing the challenges posed by music recommenders' problematic behaviours and to foster a more inclusive and diverse environment for online music discovery.
The evolution of online platforms over the past decades has radically transformed the way people discover music, and nowadays thanks to social media and music streaming services listeners have access to an ever-increasing amount of tracks and artists. Within these platforms, one of the goals of recommender systems is to help users discover music without making them feel overwhelmed while exploring the huge catalogues available.However, these systems have come under scrutiny from the scientific community, policy-makers, and civil society due to their potential negative societal impact, notably with regard to issues of fairness, non-discrimination, inclusion and diversity. Algorithmic auditing has emerged as a tool to analyse the problematic behaviours exhibited by recommender systems, and to offer remedies that can limit their negative impact.
Background
The goal of the project is to demonstrate how the involvement of end-users in the auditing process can contribute to the recognition, analysis, and mitigation of problematic behaviours which may arise while discovering music.
Objectives
The focus will be on highlighting how recommender systems, by influencing the discoverability of music, can impact listeners' exposure to culturally diverse content. In essence, AA4MD will provide:
1. Qualitative and quantitative insights into how users experience problematic behaviours of RSs when discovering music.
2. Web-based auditing tool that enables large-scale audits, developed following human-centred design practices.
3. Recommendations for policy-makers to enhance the discoverability of culturally diverse content on online platforms.
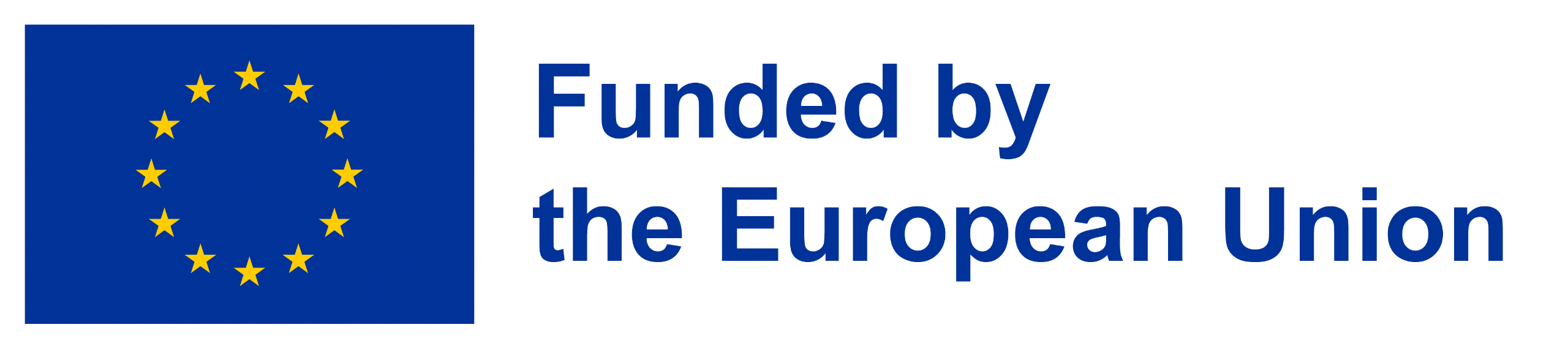
Funded by the European Union within the MSCA project AA4MD (GA n. 101148443). Views and opinions expressed are however those of the author(s) only and do not necessarily reflect those of the European Union or ERCEA. Neither the European Union nor the granting authority can be held responsible for them.Start date: 01/12/2024
End date: 30/11/2026
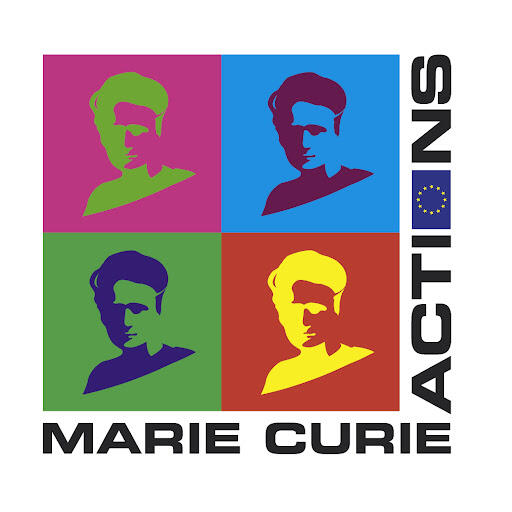
If you want to know more about the project, you can start by checking the research roadmap we presented at the 2nd Music Recommender Systems Workshop 2024, co-located with the 18th ACM Conference on Recommender Systems (RecSys 2024):Porcaro, L., Gómez, E., & Catarci, T. (2024). End-user Algorithmic Auditing for Music Discoverability: A Research Roadmap.